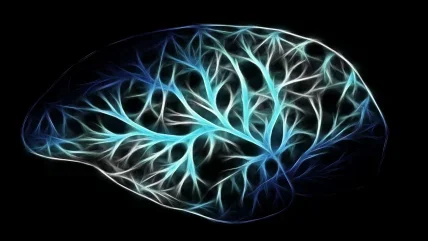
For patients with brain tumours, the right kind of surgical procedure can make all the difference. In cases where the prognosis is poor, surgeons would want to focus on maximising quality of life. They would not risk excising more tissue than necessary, leaving the patient with potentially debilitating side effects. In other types of cases, the goal would be to maximise the chances of recovery, even if that means a more intensive procedure.
Unfortunately, it isn’t usually possible for surgeons to make that call with any certainty. Because they don’t typically perform a biopsy beforehand (that would delay the process by weeks), pathologists can only analyse the tissue once surgery is already under way. And because of the complexities inherent in this process – there are around 80 different brain tumour subtypes, each with a different molecular profile – it takes a week or more to reach a diagnosis.
“If they’re not quite certain, surgeons are usually a bit more conservative in what they do during surgery, but in some cases that means the patient will need to have a second surgery later on,” explains Bastiaan Tops, head of the Laboratory for Paediatric Oncology at the Princess Máxima Center in Utrecht, the Netherlands. “Or you did too much – you excised everything, while in hindsight, you could have been more conservative.”
Together with collaborators at UMC Utrecht, Tops has been working on an AI-based solution that can dramatically speed up diagnosis. Sturgeon, the algorithm in question, can identify the tumour subtype within 20 to 40 minutes – fast enough for the surgeon to adjust their strategy mid-procedure.
“The algorithm is now being used in practice around two to three times a week,” says Jeroen de Ridder, research group leader within UMC Utrecht and the Oncode Institute. “If you think about it, that’s terrible because that means there are three paediatric brain surgeries every week in a small country like the Netherlands. But we hope that with this algorithm, we can provide the surgeon with more information to make solid decisions.”
Building on what’s gone before
The research builds on work by a team in Oslo, which used nanopore sequencing – a method for analysing DNA in real time – to classify brain tumours. By looking at methylation, genetic modifications in the DNA that vary depending on the subtype, they were able to make accurate diagnoses very rapidly. Reading their paper, Tops had a hunch it might be possible to go one step further.
“The Oslo team built a specific algorithm for each specific patient, based on the data they sequenced at that point in time,” he explains. “The problem with that is that you need to retrain an algorithm during surgery, which takes computing time, and you can never validate that algorithm because it was trained only on those specific data.”
He approached De Ridder’s team with a question: might it be possible to create a single, patientagnostic algorithm? They set to work on a solution that can be used for any patient and requires only a small amount of sequencing data.
“If I show you an image of, say, an elephant, but I only give you 1% of the pixels in the image, how can you see the elephant?” says De Ridder. “That’s what we solved with the AI. We don’t know beforehand which part of the genome we are measuring, and we know we only have time to measure a tiny fraction of it. So we have made an AI that doesn’t care which data it receives, as long as it gets a little bit – it can still make a robust decision.”
The algorithm was trained using microarray data available in public repositories and validated using data from the Princess Máxima Center biobank. De Ridder’s team ran the algorithm through the data over 30 million times before retrospectively applying the model to 50 brain tumour samples, 45 of which were classified accurately within 20–40 minutes.
“It’s kind of a black box – we don’t know exactly what the algorithm sees or what the decision is based on,” says Tops. “That means training and thoroughly validating the algorithm is of crucial importance. Thanks to the biobank samples, we now have a pre-trained algorithm that we can run in minutes, which can be continuously validated to see how well it performs.”
“It’s kind of a black box – we don’t know exactly what the algorithm sees or what the decision is based on. That means training and thoroughly validating the algorithm is of crucial importance.”
Bastiaan Tops, Laboratory for Pediatric Oncology
Although the algorithm is now being used successfully across both paediatric and adult brain surgeries, there is still a place for a human pathologist. He or she will examine the sample through the microscope and communicate their findings to the surgeon, who will interpret both sets of results as a package deal.
Opportunities and risks
While it’s still early days for this research, and larger studies are needed to assess its impact on patient outcomes, the work is generating a buzz. Simon Williams, a neurosurgical trainee who was not involved in the research, describes the paper as “excellent”.
20 – 40
The time, in minutes, taken to identify tumour subtypes during surgery by the AI-based algorithm.
Sturgeon
“It showcases exactly how AI can positively impact our field, by reducing diagnostic times from days to minutes,” says Williams, who was previously a clinical research fellow in neurosurgery, AI and robotics at the Wellcome/EPSRC Centre for Interventional and Surgical Sciences (Weiss), University College London. “The surgical implications for this alone are far-reaching. Intraoperative tissue diagnosis has been a focus and goal for surgeons and pathologists alike for many years, and the ability of Sturgeon to conduct real-time tumour sequencing advances this paradigm.”
In Williams’ view, neuro-oncology is ideally suited to AI integration. Not only is the field awash with data but there is a vast unmet clinical need, with patient outcomes largely unchanged over the past few decades. His team at Weiss have developed an AI platform that identifies relevant anatomy in pituitary tumour surgery, an area where numerous critical structures are at risk of damage. So far, this platform is proving to be extremely useful to neurosurgeons, particularly those in training.
“We are hopeful that incremental changes brought about by AI – in the areas of clinical decision support, risk stratification, and researching novel treatments – will improve outcomes for patients in the coming decades,” he says.
The caveat here is that AI is no silver bullet – it is unlikely to change outcomes for brain tumour patients overnight. As it gains more of a stronghold, we may see the emergence of poorly trained AI platforms that don’t work well with real-world data or contain an inbuilt bias. Then there’s the issue of trust – without a proper understanding of how predictions are made, clinicians may justifiably ask “Why should I trust you?”
De Ridder agrees that, as exciting as these technologies are, we need to be careful not to get swept away by the hype. “We shouldn’t treat them like magic, because they clearly are not,” he says. “Let’s take it slowly and not overestimate their utility until we know for a fact that they’re safe.”
That said, Williams thinks that there are ways of mitigating these concerns, not least by ensuring that all AI-assisted decisions are sense-checked by clinicians and that monitoring strategies are put in place for new technologies. He suspects that countries like the UK and the Netherlands are about five to ten years away from AI integration that all clinicians will palpably experience in their day-to-day lives.
“Close collaboration between innovators, regulators, clinicians and managers will be essential in ensuring that a balance is struck between ensuring appropriate evaluation and avoiding stymying progress,” he says.
Going forward, the Dutch team plans to refine their algorithm still further, training it on additional data that have become available since their initial research. They also hope to apply a similar approach to other cancer types.
“There are lots of other tumour types in which having to wait a week for diagnosis is very burdensome for the patient,” says De Ridder. “This approach could be used across any type of cancer where methylation is a good biomarker for tumour variant, such as soft tissue sarcoma.”
The technology has another key advantage, in that the equipment used is very affordable. You need a high-performance laptop (around €1,500), a nanopore sequencer and chips that can be reused several times. That’s vastly cheaper than existing microarray technologies, which often require machines worth hundreds of thousands of euros along with chips that can only be used once.
“We have already had some interest from low and middle-income countries to use these types of diagnostics,” says Tops. “These countries usually lack the equipment to get a specific molecular diagnosis, but with nanopore sequencing, it’s a much lower investment. That’s perhaps where we will have the most impact on achieving better outcomes for children and adults with a brain tumour.”