Information detailing physical and mental health is sensitive by nature, which makes it difficult for medical centres to share such vital patient data with researchers.
Exclusion from such data can limit what researchers in the field of pathology are able to achieve as they attempt to study and develop treatments for disease.
However, the artificial intelligence industry could help address privacy concerns surrounding the use of data in research projects.
Nvidia business development manager for healthcare and life sciences Craig Rhodes said: “This is very much at the forefront of the minds of everyone in the clinical healthcare domain.
“Patient records will not become more widely available — what will become available is the knowledge within those records to build intelligent models about specific disease areas that will help and support the clinical diagnosing process.
“The healthcare industries governance bodies will need to keep up with the rate of change — this will be a barrier that needs to be overcome.”
Rhodes believes there are three key areas in which patient data can be made accessible for research in a safe and secure way: Federated learning, black boxes and patient opt-in models.
Federated learning – the future of sharing patient data using AI
Federated learning is a new framework for AI model development that allows users to see the algorithm being applied to a data set, but not the data itself.
It creates a centralised deep neural network (DNN) — a machine learning structure — and each component of this network then trains its own local AI model to aggregate its data. In the healthcare research setting, these components are the various individual medical organisations.
The aggregated data is periodically submitted to a shared parameter server, which accumulates and further aggregates the information it receives, building a universal model and sharing it back to all the components of network.
This means the patient data never actually leaves the domain of the hospital or doctors’ surgery, but researchers are able collaborate on the same model without directly sharing any clinical data and compromising patient privacy.
A study by computer gaming company Nvidia and King’s College University in London found federated learning systems “can provide rigorous privacy protection with only a reasonably small cost in model performances”.
The study, which used medical imaging to identify brain tumours, also found that federated learning has the potential to aggregate data effectively, and can provide data-driven precision medicine on a large scale.
Nvidia’s Rhodes believes while other solutions bring data to a central location before developing models, federated learning means there can be more security, and consideration for ethical and governance restrictions.
He said: “Federated learning is being used in a number of organisations — it is now starting to be more widely considered as an alternative approach to building large-scale data lakes [a system in which data is stored in its natural format].”
“It’s really starting to take off because of the privacy and ethical concerns — there is a requirement to get some of these algorithms into clinical practice as quickly as possible.”
The issue of decoding ‘black boxes’ of patient data
In the simplest terms, a black box is a device or system with an input and output of data, but that offers no information about its inner workings i.e. how it does this.
Because of this, black boxes used in healthcare centres across the world contain a wealth of untapped knowledge about patients, diseases and drugs.
Rhodes believes decoding the information in these devices to reveal what they have learned will also be important in the future of medical research.
He added: “The clinical model contains the knowledge and intelligence it has learned from the clinical data that has been fed into it.
“This then becomes an invaluable source of knowledge and is used to support the clinical decision making process.”
However, while opening up black boxes can give researchers a better idea of how these types of AI and machine learning systems come to conclusions, this presents a new problem due to the sensitive nature of the data.
Rhodes said: “One key aspect of producing these clinical models is ensuring that no patient-identifiable data is contained in the model — otherwise it can be found if someone wanted to look for it.
“The models should take the knowledge and intelligence from the clinical data and use that to build the models, not the clinical data itself.
“It’s a subtle difference, but a hugely important one from a patient privacy perspective.”
PathLAKE: The research project attempting to improve patient opt-in models
In the UK, patient information can only be used as data for a research project with the individual’s explicit consent.
Rhodes said: “Patients in the later stages of life due to cancers or neurological diseases are usually happier for you to use their data — but of course you still need their specific approval.”
Under the current system, patients can only consent to their data being used in a specific research study or set of trials, as opposed to it being available for any project.
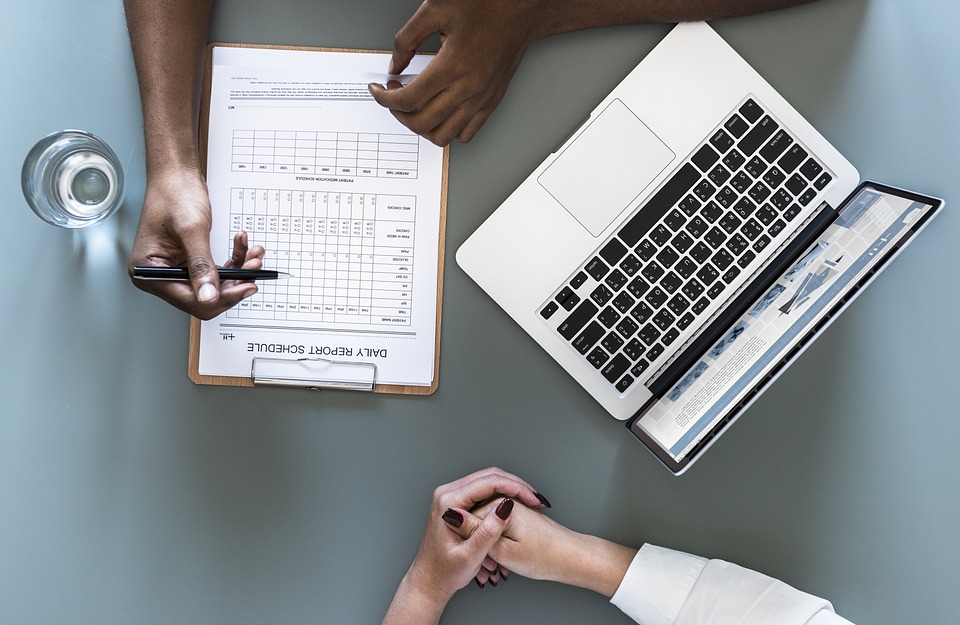
But PathLAKE, a pathology and medical imaging consortium led by the University Hospitals Coventry and Warwickshire NHS Trust, has been trialling a new model to see if the current opt-in system can be improved.
The PathLAKE project intends to create a data lake and use AI techniques to speed up the diagnosis and treatment of cancer.
Its data lake will consist of information, specifically cancer samples, taken from patients across the UK.
Rhodes said: “What PathLAKE is looking to achieve is to have patients consent to their data being used for many studies within the PathLAKE project.
“This stops the need for requesting consent over and over again for different studies.”
He added that the next step is to educate clinical professionals about how these models are being developed so they can deploy them.