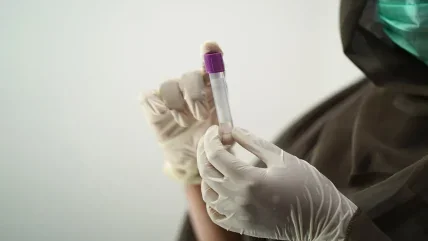
The statistics are startling: one sepsis-related death occurs somewhere in the world every 2.8 seconds. That’s 11 million preventable deaths each year and as many as 50 million infections – many of them hospital-acquired. Sepsis (or septicaemia) is a severe immune response to infection, resulting in septic shock and organ failure. It can affect anyone with an infection, meaning it can develop across diverse patient groups and clinical settings.
The Global Sepsis Alliance estimates that early detection can cut mortality rates by as much as 50%. One study found that every hour between emergency department admission and antibiotic administration can lead to a 9% increase in the chance of mortality. While prevention – including good hygiene and vaccination programs – can cut incidence rates, early diagnosis and treatment is still the last line of defence. Cutting incidence rates is easier said than done. While sepsis may be a common condition, it’s notoriously difficult to diagnose. Onset and progression are rapid and there is no single symptom or diagnostic marker that raises a red flag. In the US, sepsis has an average hospital mortality rate of 17%, reflecting both the speed at which the condition advances and frequent delays in diagnosis. Back in 2018, Augusta Health, a community hospital in Virginia, recognised that there was opportunity for improvement. Sepsis was leading to unnecessary deaths and soaking up vital healthcare resources. It was time to act.
4.76%
Average mortality rate of sepsis at Augusta Health, compared with the Virginia state average of 12.7%.
Augusta Health
A new level of support
Enter Penny Cooper and her small team of data scientists, who devised an artificial intelligence (AI)-based early alert system to identify highrisk patients. They started by taking the four main systemic inflammatory response syndrome (SIRS) indicators: high temperature (>38°C), heart rate (>90 beats per minute), respiratory rate (>20 breaths per minute) and an abnormal white blood cell count. They used these benchmarks as their key variables before adding mean arterial pressure and shock index to further increase accuracy.
Based on the results of their retrospective study, Cooper’s team then developed an algorithm to assess the key indicators every hour, automatically extracting data from the hospital’s Rauland bed system and combining it with clinical data from electronic medical records (EMRs). The system assesses the entire patient population – regardless of disease state – and assumes that every patient may be at risk of sepsis.
From there, the data is analysed and concurrent symptoms identified, with each patient allocated a ‘score’ based on the data. If a patient receives a score above a predetermined cut-off point, an alert is triggered and sent to nursing staff. Augusta Health uses Vocera communication devices, but Cooper says alerts could be routed to any similar system, or indeed to mobile phones or emails.
The system – introduced into the emergency department in August 2018 and then to the wider patient population two years later – has cut the hospital’s sepsis mortality rate to 4.76% compared with the Virginia state average of 12.7%. Cooper estimates more than 300 lives have been saved when predicted mortality is compared with actual mortality.
Simplicity is the key
The beauty of the system lies in its simplicity – feeding the right data to the right algorithm and letting AI do the rest. Staff don’t need to spend any extra time collecting data – it is all already captured during assessment and treatment. Moreover, instead of clinicians analysing and comparing the data to identify which patients are at risk of sepsis, the system does it for them. Automating the process also helps eliminate concerns over privacy or consent. “The data is being collected in the EMR anyway and the nurses we’re alerting are the nurses who are directly attending that patient – we’re not sending that alert out to a different nurse,” says Cooper. “The alert is also only sharing basic information – a room and bed number – it doesn’t even include the patient’s name.”
Cooper’s team has also adapted the system for different clinical settings, changing the parameters in line with available data. More variables are included for emergency department (ED) patients than for inpatients, for example. Some variables are also excluded to reduce error. “White blood count is not included for inpatients because labs are only taken once a day and we didn’t want old lab values to impact the current results,” explains Cooper.
She insists the alert system was not difficult to set up, nor was it expensive. The algorithm was devised and programmed in-house, without the use of external consultants or software developers. “We’re a pretty small shop,” she says of Augusta Health, which has just 255 beds. The hospital’s data team is made up of Cooper and three other analysts. “The actual technical implementation really didn’t take that long – just a few months – but what we took our time on was making sure that we ran it extensively in the background to check the variables and to ensure the nurses weren’t going to be overwhelmed by the number of alerts.”
She stresses the importance of not only data availability, but also the need to use it effectively. “It’s a fine line when you are dealing with so much data – it’s precision medicine at that point,” she says. “It’s like dumping everything into a bucket and seeing what’s what. There are probably other variables we could go back and add – like alert status and SOFA [sequential organ failure assessment] scores to evaluate a patient’s deterioration, but for the moment our system is working. If it ceases to work, we can go back and add other variables to the mix.”
Wealth of opportunities
While Augusta Health isn’t the only institution using AI and machine learning to fight sepsis, it is one of the smaller operations – and Cooper is adamant that data analysis technology can be implemented by health providers of all sizes.
Cooper says Augusta’s findings have already been shared with other hospitals in Virginia – both large and small – with a view to ensuring more lives are saved. “There’s no reason why other small community hospitals like ours cannot use a system like this,” she says. “You’re selecting key data elements during an initial analysis and then pulling the latest instances of those vitals – respiration, pulse, temperature – for every patient and evaluating that based on set parameters. It’s a very simple process.
“I think my advice would be: don’t let the data intimidate you because it’s not overly difficult once you break it apart. There is so much opportunity to improve the outcome for patients and to improve the environments that our physicians and nurses work in.”
Data engagement is also vital – and something that has been extremely positive at Augusta Health. Cooper’s team has had input from across the hospital, from pharmacists and emergency room nurses to management. Clinical and administrative staff recognise that AI-led systems can not only improve patient outcomes but also free up valuable time and expertise. That buy-in has been crucial. “As the data team is non-clinical, we need that input to guide us,” says Cooper, who at the time of writing had turned her attention to Covid projections.
She says data is vital to modern and efficient healthcare systems, but AI and machine learning are gradually removing layers of manual functions to make more efficient use of staff time and assist with faster, more accurate record keeping and diagnosis.
“There is definitely a lot of opportunity, but one of the biggest problems in terms of getting systems like this fully automated is our documentation,” Cooper says. “The data needs to be stored in discrete data elements and not free text. When it’s stored in discrete data elements, a date, for example, looks like a date and is evaluated as a date.” Without discrete data elements, Cooper says she’s seen some “very messy data”.
As clean and consistent as the data they’re fed might be, all AI-based systems must come with clear caveats. “We make sure that when we push out data to users that this is not a replacement for good clinical judgement,” she says, stressing that the system acts as another diagnostic tool – it does not replace or replicate nurse or physician decision making.
Cooper says diagnostic AI algorithms like the ones devised for sepsis could be adapted across other disease states, potentially making a whole raft of conditions easier to identify and treat. Indeed, the system could also be designed to identify disease states that aren’t even on a clinical team’s radar. If you looked at diabetes, Cooper says, and used the information from lab results in combination with patient obesity levels and other social determinants, then you could identify which patients are at a high risk for the disease. The algorithm could even be adapted not to predict disease state, but the likelihood of readmission to the hospital. “It’s really not rocket science”, says Cooper.
Using AI to predict cancer
Sepsis isn’t the only disease state that’s predictable with AI. Researchers are coming up with different algorithms all the time to try and get a head start on one of the world’s biggest killers – cancer. The below are just two examples of how AI is being applied in the field of oncology.
Lung cancer – Researchers from the Diagnostic Image Analysis Group at Radboud (DIAG) University Medical Centre (RUMC) in Nijmegen developed a deep learning algorithm for malignancy risk estimation of pulmonary nodules detected at low-dose screening CT scan. When they evaluated it by pitting its predictive capabilities against experts in the field of radiology and pulmonology, they found it performed with equal accuracy, meaning it could be a valuable way to triage patients by risk. The researchers plan to continue improving the algorithm by incorporating clinical parameters like age, sex and smoking history.
Pancreatic cancer – AP-HP Greater Paris University Hospitals, the European clinical trial centre with the largest amount of healthcare research data in France, and Owkin, a start-up harnessing AI for medical research and clinical development, created a tool that uses deep learning models to identify pancreatic adenocarcinoma molecular subtypes from histology slides. Pancreatic adenocarcinoma is complex due to its heterogeneity and tumour plasticity – the ability of cancer cells to adapt to gain therapeutic resistance.
The tool, HE2RNA, connects information at the genomic, cellular and tissue levels to determine whether tumours are basal-like or classical – the two transcriptomic subtypes proposed to be predictive of patient response to chemotherapy. The tool is currently marketed for use in clinical trials for pancreatic cancer treatments.